The task deals with the automatic detection of manipulation and misuse in Web multimedia content. Its aim is to lay the basis for a future generation of tools that could assist media professionals in the process of verification. Examples of manipulation include the malicious tampering-doctoring of images/videos, e.g., splicing, removal/addition of elements, while other kinds of misuse include the reposting of previously captured multimedia content in a different context (e.g., in a new event) claiming that it was captured there. Figure 1 illustrates two real-world examples of such practices.

Figure 1: Examples of malicious Web multimedia: a) digitally manipulated photograph of an IAF F-16 deploying a single flare over Southern Lebanon; the flare was digitally duplicated to make it appear that several missiles were being fired; b) a photograph that is a repost from a 2009 art installation.
The definition of the task is the following: "Given a tweet and the accompanying multimedia item (image or video) from an event that has the profile to be of interest in the international news, return a binary decision representing verification of whether the multimedia item reflects the reality of the event in the way purported by the tweet."
The task will also ask participants to return an explanation (which can be a text string, or URLs pointing to resources online) that supports the verification decision. As explained below, the explanation will not be used for calculating the evaluation metric, but rather for gaining insights into the results.
Target group
This task targets researchers from several communities including multimedia, social network analysis, computer vision, and natural language processing. Though the task can be tackled by multimodal approaches, we welcome also approaches based on individual modalities (e.g. text-based). To help prospective participants and lower the barrier to participation, we plan to release resources that could be useful for the task (e.g., text and visual features).
Data
The data set will be a set of Twitter identifiers of tweets associated with multimedia items, and the corresponding multimedia item URLs that were shared through these tweets. An initial data set has already been created (Boididou et al., 2014), and is publicly available on GitHub: https://github.com/MKLab-ITI/image-verification-corpus. Overall, there are currently ~400 images that are used in about ~20K different tweets in the context of ~10 events (Hurricane Sandy, Boston Marathon bombings, etc.).
In order to ensure that all participants have the same data set, we will follow a “Download window” procedure. Note that we do not distribute either the tweets or multimedia items directly, but rather only links. For this reasons, the participating teams must crawl the material as well. We will declare a “Download window” of 2-3 days during which the teams agree that everyone will download the data. Any data that is not downloaded by everyone will be eliminated from the official data set. To help participants focus on the actual data analysis, we will make available scripts that take care of the necessary crawling/data collection operations.
Ground truth and evaluation
Overall, we are interested in measuring the accuracy with which an automatic method can distinguish between use of multimedia items in tweets in a way that faithfully reflects reality and in a way that spreads false impressions. Hence, given a set of labeled instances (image + context + label) that could be used for training, the participants should predict the labels of the test cases. Classic IR measures will be used (i.e., P-R or F-score) in order to quantify performance.
The task will place emphasis on gaining insight into the features and techniques that do and do not work. For this reason, we will encourage participants to design their methods in such a way so that the automatically produced verification decisions (real/fake) are accompanied by an automatically generated explanation. This could consist, for instance, of a few words or a URL. However, this output component will be optional and will not be used by the ranking process. Yet, this information will be helpful for determining which approaches function best, and also where the toughest challenges lie that should be faced in future work.
Recommended reading
[1] Boididou, C., Papadopoulos, S., Kompatsiaris, Y., Schifferes, S., Newman, N. Challenges of computational verification in social multimedia. In Proceedings of the companion publication of the 23rd international conference on World wide web companion (WWW Companion '14), pp. 743-748
[2] Conotter, V., Dang-Nguyen, D.-T., Riegler, M., Boato, G., Larson, M. A Crowdsourced Data Set of Edited Images Online. In Proceedings of the 2014 International ACM Workshop on Crowdsourcing for Multimedia (CrowdMM '14). ACM, New York, NY, USA, 49-52
Task organizers
Symeon Papadopoulos, CERTH-ITI, Greece
Christina Boididou, CERTH-ITI, Greece
Katerina Andreadou, CERTH-ITI, Greece
Giulia Boato, U. Trento, Italy
Duc-Tien Dang-Nguyen, U. Trento, Italy
Task auxiliaries
Michael Riegler, Simula, Norway
Martha Larson, TU Delft, the Netherlands
Task schedule
1 May Development data release
10 July Test data release
14 August Run submission
21 August Results returned to participants
28 August Working notes paper deadline
14-15 September MediaEval 2015 Workshop
Acknowledgments
This task is supported by the REVEAL EC FP7 Project.
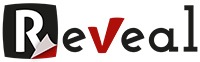
Check out their informative website: http://revealproject.eu