“Emotion in Music” is a task on time-continuous estimation of emotion in music. The emotional message of the music can change within the same piece of music, and it is a fundamental challenge to estimate the emotional character of music in a time-continuous fashion. The Valence-Arousal model will be employed to describe changes in music on two orthogonal axes. This is the third year that the task is running, and there is a large amount of data available. This year we will focus on annotation quality. We will select best quality annotations (from existing 1744 songs) from two previous years for the development set, and annotate 250 more excerpts for the test set. The musical excerpts of 45 seconds and annotations will be released to participants. All the music is licensed under Creative Commons.
The “Emotion in Music” 2015 task will comprise three subtasks. Participants will be asked to estimate and submit the Arousal and Valence scores continuously in time for every music piece in the test set in three required conditions:
- Using a fixed feature set provided by the organizers and the regression models of their choice;
- Using a fixed regression model; multiple-linear regression. The feature set should be submitted as well.
- Using both feature sets and regression models of their choice.
Teams are allowed to submit 5 runs in total for all subtasks. However, they are obliged to submit at least one run for each of the three subtasks. The goal of subtask 1 is to find the best regression approach independently of the feature set used. The goal of subtask 2 is to find the best feature set for the time-continuous estimation of arousal and valence independently of the model used. Finally, subtask 3 will determine the best global approach.
For each submitted feature set from subtask 2, the organizers will create two linear regression models that estimate the time-continuous arousal and valence scores using the development set. Then, the primary metric of the task, Root-Mean-Square Error (RMSE), will be computed on the test set to estimate the performance of each feature set for arousal and valence (separately).
The proposed sets of features can consist of new, existing or a combination of both, but all the features have to be reproducible. The code that can regenerate the features should be submitted together with the runs.
Target group
Researchers in the areas of music information retrieval, music psychology (music perception and cognition, music and emotion), speech technology, affective computing, and mathematical and computational modeling.
Data
As compared to previous year, our dataset will be smaller, but with higher quality. We will select a subset of 1744 songs used last year and release them as development set. Then, we will annotate 250 more songs as testset.
Ground truth and evaluation
The ground truth is created by human annotators and is provided to participants by the task
organizers. For all the subtasks RMSE will serve as the primary metric, and concordance correlation coefficient (ccc) as the secondary one.
Recommended reading
[1] Soleymani, M., Caro, M. N., Schmidt, E. M., Sha, C. Y., & Yang, Y. H. (2013). 1000 songs for emotional analysis of music. In Proceedings of the 2nd ACM international workshop on Crowdsourcing for multimedia (pp. 1-6). ACM.
[2] Kim, Y. E., Schmidt, E. M., Migneco, R., Morton, B. G., Richardson, P., Scott, J., & Turnbull, D. (2010). Music emotion recognition: A state of the art review. In Proc. ISMIR (pp. 255-266).
[3] Yang, Y. H., & Chen, H. H. (2012). Machine recognition of music emotion: A review. ACM Transactions on Intelligent Systems and Technology (TIST), 3(3), 40.
[4] Barthet, M., Fazekas, G., & Sandler, M. (2013). Music Emotion Recognition: From Content-to Context-Based Models. In From Sounds to Music and Emotions (pp. 228-252). Springer Berlin Heidelberg.
[5] Coutinho, E., & Dibben, N. (2013). Psychoacoustic cues to emotion in speech prosody and music. Cognition & emotion, 27(4), 658-684.
[6] Weninger, F., Eyben, F., Schuller, B. W., Mortillaro, M., & Scherer, K. R. (2013). On the acoustics of emotion in audio: what speech, music, and sound have in common. Frontiers in psychology, 4.
Task organizers
- Anna Aljanaki, Utrecht University, the Netherlands (a.aljanaki@uu.nl) Anna is a digital music researcher and a musician. She has been instrumental to the task since last year and is the leading organizer this year.
- Mohammad Soleymani, University of Geneva, Switzerland (mohammad.soleymani@unige.ch) Mohammad is one of the founding organizers of MediaEval, and has designed and organized tasks since the beginning of the benchmark.
- Yi-Hsuan Yang, Academia Sinica, Taiwan (affige@gmail.com) Eric has been an organizer since 2013 and an established researcher in the field of Music Information Retrieval. He was one of the program chairs of ISMIR 2014.
Task auxiliary
Alexander Lansky, Queens University, Canada
Task schedule
19th of May Development data release (updated)
31 July Test data release
15 August Run submission
28 August: Working notes paper deadline
14-15 September MediaEval 2015 Workshop
Acknowledgments
The work of Anna Aljanaki is supported by COMMIT/.
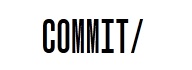