MediaEval 2019
MediaEval is a benchmarking that offers challenges in multimedia retrieval, access and exploration. Our mission is to allow researchers working in computer science and other multimedia related field an opportunity to work on tasks that are related to human and social aspects of multimedia. MediaEval emphasizes the 'multi' in multimedia and seeks tasks involving multiple modalities, e.g., audio, visual, textual, and/or contextual. Our larger aim is to promote reproducible research that makes multimedia a positive force for society.
Thank you everyone for a successful and interesting workshop. A very special word of appreciation to EURECOM for hosting us. Workshop materials can be found here:
- MediaEval 2019 Workshop Proceedings
- MediaEval 2019 Workshop Program
- Presentation videos: MediaEval Community YouTube Channel
- Slides: http://www.slideshare.net/multimediaeval
- For pictures, check out MediaEval on Flickr
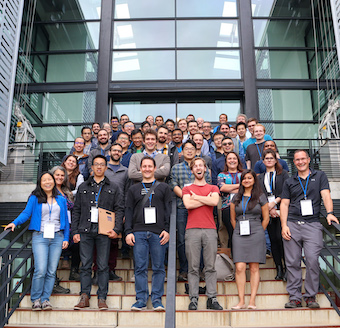
Workshop Schedule
Sunday 27 October
14:00 Kickoff (Location to be announced. It will be near EURECOM, Sophia Antipolis, France)
Evaluation methodology session (all tasks)
17:00 Hike to restaurant (4 km weather permitting) (Alternative transportation for people who would not like to hike.)
18:00 Gentle walk in the medieval village of Biot and then Workshop Dinner
Monday 28 October
EURECOM, Sophia Antipolis, France
9:00-12:00 Workshop
12:00-14:00 Lunch
14:00-19:00 Workshop
Workshop Dinner
Tuesday 29 October
EURECOM, Sophia Antipolis, France
9:00-12:00 Workshop
12:00-14:00 Lunch
14:00-18:30 Workshop
18:00 Distinctive Mentions and workshop close
Wednesday 30 October
Bonus day (Stay for this day if you are interested in evaluation methodology, if you are planning to organize a task in 2020, or if you working on disaster management research, and would like to present and/or discuss MMSat and plan for next year.)
EURECOM, Sophia Antipolis, France
9:00-10:00 Work session/MMSat project presentations
10:00-12:00 Outlook: Presentations of plans for next year
12:00-14:00 Lunch
14:00-15:00 Work sessions/MMSat further planning
MediaEval 2019 Timeline
April-June 2019: Registration for task participation
May-June 2019: Development data release
June-July 2019: Test data release
Run submission: mid-September 2019
Working notes paper deadline: 30 September 2019
Workshop: 27-29 October 2019 near Nice, France (scheduled so that participants can combine the workshop with attendance at ACM Multimedia 2019 in Nice).
Task List
The list of tasks offered in 2019 is below. Register to participate on the MediaEval 2019 registrations website. Once you have registered, please fill in the MediaEval 2019 Usage Agreement and return it following the instructions on the first page.
Emotion and Theme recognition in music using Jamendo
The goal of this task is to recognize the emotions and themes conveyed in a music recording. A common approach involves predicting the tags (e.g. happy, sad, melancholic) that describe it. To build the dataset for this task we use a collection of music from Jamendo that is available under the Creative Commons license with tag annotations that come from uploaders. The evaluation will be performed using the traditional metrics of prediction accuracy. Read more...
Eyes and Ears Together
Participants of this task are expected to build a system that analyzes video (visual features and speech transcripts) and creates bounding boxes around the regions of the video frames that correspond to nouns and pronouns in the speech transcript. The dataset used for the investigation is a collection of instruction videos, the How2 dataset. The output of participant systems will be evaluated in terms of the accuracy of the bounding boxes. The task is designed to encourage researchers to work on textual and visual domains simultaneously, and to advance research on multimodal processing. Read more...
GameStory: Video Game Analytics Challenge
In this task, participants analyze multi-view, multimedia data captured at a Counter-Strike: Global Offensive event. The data includes sources such as audio and video streams, commentaries, game data and statistics, interaction traces, viewer-to-viewer communication. We ask participants to develop systems capable of multi-stream synchronization, replay detection, and ultimately: summarization towards a GameStory. The task opens the area of e-sports (which has over 150 million regular users) for multimedia researchers. Read more...
Insight for Wellbeing: Multimodal personal health lifelog data analysis
Participants receive a set of weather and air pollution data, lifelog images, and tags recording by people who wear sensors, use smartphones and walk along pre-defined routes inside a city. The “segment replacement” subtask requires participants to develop a hypothesis about the associations within the data and build a system that is able to correctly replace segments of data that have been removed. The “AQI prediction” subtask requires participants to predict AQI (Air Quality Index) using either the underspecified data or full data from a subset of data sources. The data are collected from the "datathon" campaign that took place in Fukuoka city, Japan in 2018 and 2019. Read more...
Medico Medical Multimedia
The goal of the task is the efficient processing of medical multimedia data for sperm quality prediction. Task participants are provided with a multimodal dataset (videos, analysis data, study participant data) in the field of human reproductive health. The task will be to predict the motility (movement) and morphology (shape) of spermatozoa. The subtasks will focus on the different modalities contained within the dataset, and how they may be combined. The ground truth was created through a preliminary analysis done by medical experts according to the World Health Organization’s standard for spermatozoa quality assessment. Read more...
Multimedia Recommender Systems
Participants can choose between one of two tasks that investigate the use of multimedia content for the purpose of improving the ability of recommender systems to predict items relevant to users’ interests. Participants analyze items and create feature sets that combine modalities (audio, visual, image, text). The first task is movie recommendation and requires participants to predict the average rating of a movie and the variance of that rating. The movie dataset includes links to the videos (Youtube URLs), precomputed state of the art audio-visual features, and metadata from MovieLens. The second task is news recommendation and requires participants to predict the number of views for news articles. The news dataset is collected from a set of German publishers and spans multiple months. It includes text snippets, image URLs (and some pre-extracted neural image features). Read more...
Multimedia Satellite Task: Flood Severity Estimation
The purpose of this task is to combine the information from in satellite images and online media content in order to provide a comprehensive view of flooding events. The task involves three subtasks: (1) Flood severity estimation from images and newspaper articles online, (2) Flood severity estimation from satellite images and (3) Identification of images shared online that contain deceptive (“fake”) information on flooding events. Participants receive multimedia data, new articles, and satellite imagery and are required to train classifiers. The task moves forward the state of the art in flood impact assessment by concentrating on aspects that are important but are not generally studied by multimedia researchers. Read more...
No-audio Multimodal Speech Detection
Participants receive videos (top view) and sensor readings (acceleration and proximity) of people having conversations in a natural social setting and are required to detect speaking turns. No audio is signal is available for use. The task encourages research on better privacy preservation during recordings made to study social interactions, and has the potential to scale to settings where recording audio may be impractical. Read more...
Pixel Privacy
Video trailer explaining the task
Participants receive a set of images and are required to enhance them. The enhancement should achieve two goals: (1) Protection: It must block the ability of an automatic pixel-based algorithm from correctly predicting the setting (scene class) at which the photo was taken (i.e., prevent automatic inference) and (2) Appeal: It must make the image more beautiful or interesting from the point of view of the user (or at least not ruin the image from users’ point of view.) The task extends the state of the art by looking at the positive (protective) ability of adversarial machine learning, and also exploring how people’s natural preference for appealing images can be linked to privacy protection. Read more...
Predicting Media Memorability
For the task, participants will be provided with extensive datasets of multimedia content (images and/or videos) associated with memorability annotations. Participants will be required to train computational models capable to infer multimedia content memorability from features of their choice (some features provided). The ground truth consists of scores reflecting how memorable (both in the short and the long term) video content is for a general-audience viewer, which was collected using recognition tests. Read more...
Scene Change (Brave New Task)
The task is interested in exploring fun faux photo’s, images that fool you at first, but can be identified as an imitation on closer inspection. Task participants are provided with images of people (as a “foreground segment”) and are asked to change the background scene to Paris. Results are evaluated by user studies that measure how long a general-audience requires to discover that the background has been switched. The task encourages the development of technology that allows people to fantasize with photos without engaging in deceptive practices. Read more...
Sports Video Annotation: Detection of Strokes in Table Tennis (Brave New Task)
Participants are provided with a set of videos of table tennis games and are required to build a classification system that automatically labels video segments with the strokes that players can be seen using in those segments. Later years will build upon this first, basic task. The ultimate goal of this research is to produce automatic annotation tools for sport faculties, local clubs and associations to help coaches to better assess and advise athletes during training. Read more...
Task Force
Task forces are groups of people working together to design and plan a task to be offered in future years.
NewsFire: Discovering the triggers for viral news stories
Participants receive a large corpus of news stories and social media posts (text and images) and are required to build a system that detects the original triggers of news that spread with a viral or wildfire pattern. They are encouraged to develop “news graphs” in which the nodes represent content items and the edges represent topical relationship or topical influence. If you are interested in the work of a task force and would like to have more information about what is being planned, or would like to get involved, please contact Konstantin Pogorelov at konstantin (at) simula.no
If you are interested in proposing a task:
The deadline has passed for 2019 tasks. However, you can still start a Task Force and/or begin working on your proposal for next year. Please contact Martha Larson at m.a.larson at tudelft.nl
General Information about MediaEval
MediaEval was founded in 2008 as a track called "VideoCLEF" within the CLEF benchmark campaign. In 2010, it became an independent benchmark and in 2012 it ran for the first time as a fully "bottom-up benchmark", meaning that it is organized for the community, by the community, independently of a "parent" project or organization. The MediaEval benchmarking season culminates with the MediaEval workshop. Participants come together at the workshop to present and discuss their results, build collaborations, and develop future task editions or entirely new tasks. MediaEval co-located itself with CLEF in 2017, with ACM Multimedia in 2010, 2013, and 2016, and with the European Conference on Computer Vision in 2012. It was an official satellite event of Interspeech in 2011 and 2015. In 2019, we celebrate our ten-year anniversary with a workshop held just after ACM Multimedia 2019. Past working notes proceedings of the workshop include:
MediaEval 2015: http://ceur-ws.org/Vol-1436
MediaEval 2016: http://ceur-ws.org/Vol-1739
MediaEval 2017: http://ceur-ws.org/Vol-1984
MediaEval 2018: http://ceur-ws.org/Vol-2283
MediaEval 2019 Sponsors and supporters
Intelligent Systems, Delft University of Technology, Netherlands
SIGMM ACM Special Interest Group on Multimedia

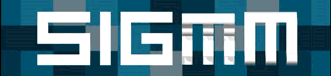
SIG SLIM: ISCA Special Interest Group in Speech and Language in Multimedia


Did you know?
Over its lifetime, MediaEval teamwork and collaboration has given rise to over 750 papers in the MediaEval workshop proceedings, but also at conferences and in journals. Check out the MediaEval bibliography.